
Alexa Woodward, PhD, MS
Partnerships and Strategy Manager, Clinicogenomics, Optum Life Sciences
Advancing discovery and treatment using genomic data
Insights unlocked from human genome data hold great power for the life sciences industry. But stand-alone, genomic data are of limited value. To truly understand clinical decisions and outcomes, you need data about the entire patient experience through the health care system.
Layering genomics data or other -omics data on top of robust clinical and claims data creates data sets that can bring researchers meaningful, fit-for-purpose information. Assessing the patient journey both before and after diagnosis is crucial to help determine effective treatment regimens, understand diagnosis and progression, and improve patient lives. The applications across the entire drug discovery and development lifecycle are broad. They include the discovery of new druggable targets to creating external control arms, post-market surveillance and beyond.
Many forward-thinking organizations are already pursuing genomics-driven studies and have successfully marketed new products based on genetically supported targets. But clinicogenomic research isn’t without its challenges.
Creating and analyzing a curated data set has many steps and checkpoints. Researchers need to make sure that the data they’re working with are analyzed in the right context and meet their stakeholders’ standards for quality information.

Researcher concerns and how Optum is bridging the gap
You may encounter several tough decision points when pursuing precision medicine and targeted treatments. Some of these factors are in your control and some of them aren’t. What’s most important is being diligent in choosing high-quality data that align with your study objectives and bring value to your research.
Availability of genomic data resources
As a form of real-world data (RWD), genomic data reflect real patient lives. As such, available genomic data are constrained by the types of tests that physicians order. And a lot of factors influence which tests they decide to order.
Physicians must consider the different tests on the market for a given condition (single variants, panels, whole exome or genome, etc.), resources available for the patient to undergo testing, health plan coverage of the tests, etc. This shows the impact that clinical decision-making can ultimately have on data sets.
Then there’s the question of overlapping patient data. You need all the pieces of the puzzle to successfully merge disparate data sources. For example, do you have cancer genomics, pan-therapeutic clinical data and medical and pharmacy claims for the same group of people with non-small cell lung cancer (NSCLC) over a defined period? Or are the data discordant — genomics from a cancer diagnosis, clinical data from a broken arm and claims data from obstetrical care?
That’s why Optum combines the rich depth of genomic sequencing data with a subset of our 115 million clinical lives.* For a wide range of therapeutic areas, researchers can get a detailed look into real-life care.
Context surrounding genomic databases
There are many situations where a patient is diagnosed in one setting and treated in another. Or maybe they were diagnosed with a different health condition before their genetic-related disease emerged that may influence care options and treatment decisions.
With a clinicogenomic data set that spans specialties and sites of care, you get a clearer picture of the patient journey. Again, think of a patient with NSCLC. Maybe the patient was first examined by a primary care physician for initial symptoms, referred to a pulmonologist, and ultimately treated and underwent genetic testing with an oncologist.
Then multiply that patient and consider a sample of similar journeys. Layered data can show you the early stages through treatment and beyond. You can’t just rely on single-specialty genomic data to understand the overall trajectory for NSCLC patients.
Other helpful context may include sequential sequencing. It’s not uncommon for patients to have results from more than one genetic test. Especially in cases such as oncology, physicians may use tests for recurrence monitoring, allowing researchers to assess factors like line of therapy and disease progression in relation to historical tests.
Our solid tumor data include sequential sequencing for over 13% of individuals, because tumor biology changes over time and in response to different treatments. More than just knowing the test results, you want to know what kind of tumor cells the first line of therapy selected against and what's driving patient progression at this point.
Cohort building: Data set size and diversity
Data sets are only useful if they reflect the real-world population that you want to study. When you start to ask very specific questions, the eligible study population can shrink quickly. And so, one of your challenges may be figuring out the best cohort to answer your questions.
Sometimes that becomes a meaningful cohort of 75 patients because they're highly characterized. Other times, there’s a lot of information about the patients, and the strong longitudinal data give you a 1,000-person cohort. The Optum clinicogenomics team can help you figure out what information you really need and where there’s relevant overlapping data.
Diversity is increasingly important in building cohorts, too. Ideally, you want data that reflect a variety of demographics. When diversity is lacking in foundational data sets, you’re missing information on how to develop diagnostics, understand disease progression and develop medications that are safe and effective for everyone. That’s why for certain therapeutic areas, you can design cohorts with our data that are 100% non-Caucasian to analyze specific demographic trends.
Genomic data in action: Colorectal cancer example
In one recent internal Optum Life Sciences study, researchers used a curated clinicogenomic data set to study demographic and clinical factors in the treatment of colorectal cancer (CRC). They wanted to determine which factors influenced who got targeted treatment after receiving genetic or genomic testing using liquid biopsy-based, broad panel next-generation sequencing (NGS).
The data revealed that those who had a genetic counseling session after receiving their test results were 65% more likely to get targeted treatment for their CRC. Survival data for those who received targeted treatment indicated that those patients almost always fared better compared to those who did not receive it. This held true even when accounting for other variables.
The data also unlocked other interesting patterns: older patients, patients living in the Midwest region of the U.S., Black patients and those treated in a community setting (as opposed to an academic setting) were all less likely to get targeted treatment.
This example unearths many rich questions: Why do the data show these patterns? What challenges does the industry face in providing patients with different demographic backgrounds the treatments they need? How can we get more patients on targeted therapies? What other characteristics about the patients who got the targeted therapy made them a good candidate for the treatment and optimized their chances of survival?
With the additional context provided by clinical and claims data — such as comorbid conditions, sites of care, signs and symptoms, other basic patient demographics — you can begin to tackle these questions. That then allows you to spend more time on productive, actionable research and development that ultimately drives impact for patients.
Clinicogenomics help catalyze research for complex conditions
Life sciences researchers are asking increasingly difficult questions to fuel their work and bring stakeholders the data and evidence they need. With clinicogenomic data, researchers have more opportunities than ever before to unpack how lifestyle, comorbidities and environmental factors influence patient outcomes.
Though the examples presented here are oncology-specific, applications of genomic data are vast. You can create cohorts from our data for inflammatory bowel disease, chronic kidney disease, type 2 diabetes, rare diseases and more.
Clinicogenomic data hold a lot of potential, and the field is only growing. And the resulting advancements could mean fewer resources deployed toward unsuccessful research and development, faster time to market and enhanced guidelines for appropriate use. Fuel your next discovery.
Discover the potential of genomic data
Interested in learning more about the value of clinicogenomic data? Contact us today.
Related content
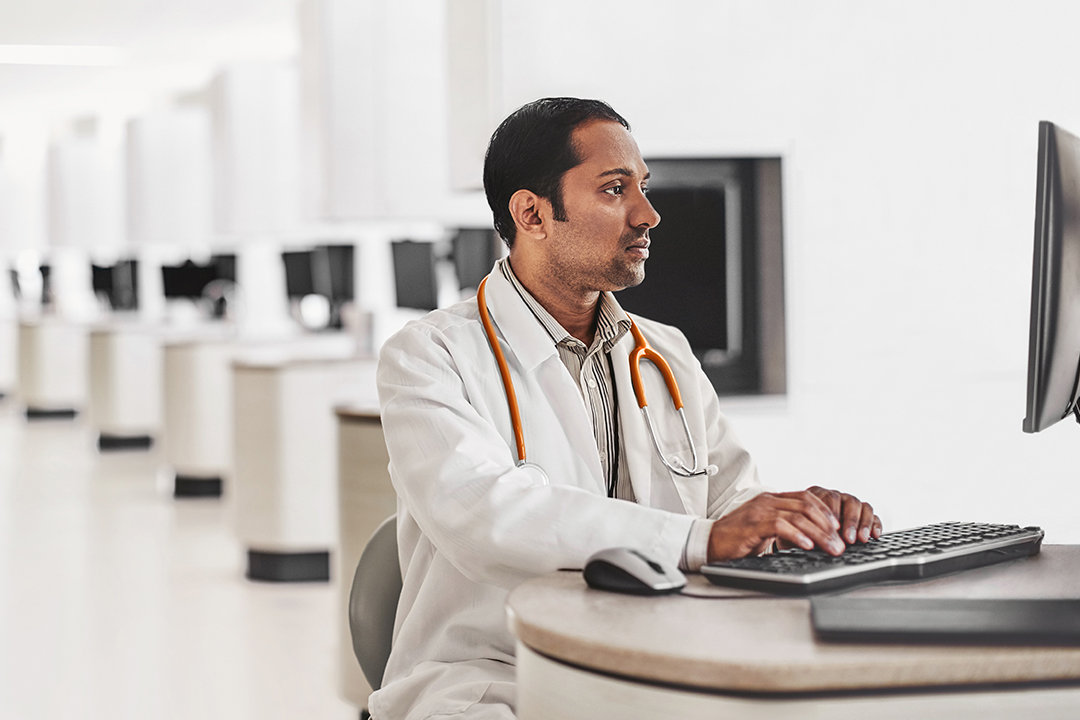
Clinicogenomics: advancing discovery and treatment

The 6 trends shaping pharma strategies in 2024
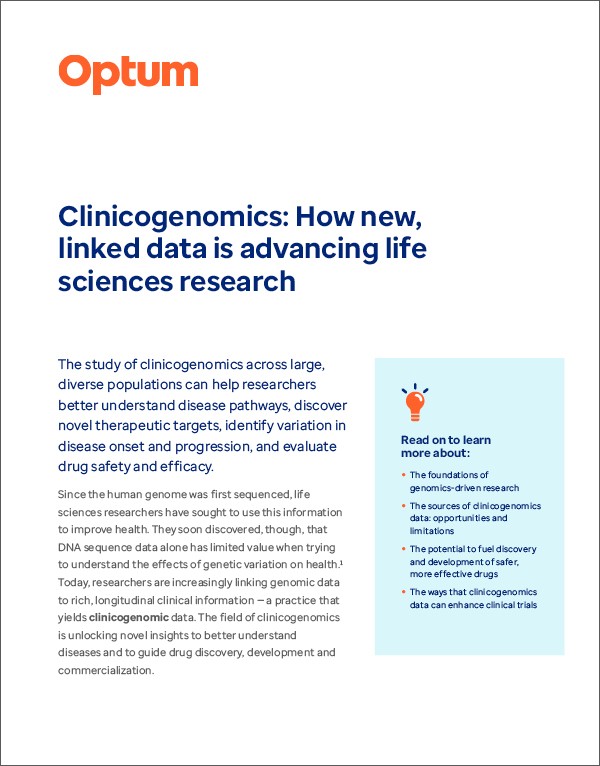
Clinicogenomics: new, linked data is advancing research
*All data are acquired and used in accordance with applicable statutes, regulations and contractual limitations.