On-demand webinar
Reach the correct patients with consumer data
Learn how consumer data can support your health system for the years ahead.

How to Reach the Right Patients with Consumer Data | Optum
[Heather]
And thank you for joining today's webinar, transforming the patient journey through big data. My name is Heather Vollmer with Optum, and I'll be your host today. Before we begin, please note the following housekeeping items. At the bottom of your audience console are multiple application widgets you can use to customize your viewing experience. If you have any questions during the webcast, you can click on the Q&A widget at the bottom of your screen to submit a question. We do capture all questions and will be providing follow-up to questions as appropriate. If you experience any technical difficulty, please click on the help widget. It covers common technical issues. You can expand your slide area by clicking on the maximize icon on the top right of the slide window, or by dragging the bottom right corner of the slide window. There is a survey widget, which you can use at the end of the webcast to provide us with feedback on today's presentation. Additionally, this presentation uses streaming audio. You may listen to the audio through your computer speakers or headphones. To ensure the best possible system performance, please be sure to shut down any VPN connections and connect directly to the internet. And with that I will hand it over to Brian.
[Brian]
Yeah, thank you. So we have two presenters today. My name is Brian Michels. I'm a senior strategist on the Optum team. I've been with Optum for, well, I was with the advisory board, and then Optum for probably the last 10 years. Worked across a couple of different products and platforms with the organization. Before coming to the advisory board, I was a director of strategy and business develop for a 12 hospital system as well. And so have used a number of different data assets and different platforms to utilize those data assets throughout my years working in healthcare. I'm joined by my partner who I'll pass it over to and have introduce himself.
[Nick]
Thanks Brian. This is Nick Connors. I'm another senior strategist here at Optum. I've spent the last 10 years or so of my career in various roles that all focus on the analytics of our national claims data assets. And so that will drive a significant portion of the discussion and sort of my section of today's presentation. And so wanted to start with a brief overview of our agenda for today. First up, we're gonna begin with the discussion on longitudinal claims data. What that means, how it differs from other available resources, what types of analysis longitudinal data can either augment or create as you think about your providers in your market, your patients. And then I'm gonna pass things back over to Brian for a discussion on available consumer data resources. He'll talk through how this data can round out your understanding of your market, as well as how that data is useful, both on its own and in combination with some of the longitudinal data that we'll discuss in the first part of today's presentation. And then we'll end our discussion today with more specific detail on how some real-world organizations are actively leveraging these two data sets in the market. We'll be keeping an eye out for questions as they come in, but Brian and I will also make sure that we leave some time at the end for Q&A in case you have questions around either these data assets, or how they might apply to specific analyses that you might have in mind for conducting within your market. So first off, let's begin with the discussion of longitudinal data, what it is and essentially how it's different. I want to start actually with a quick poll question. Do you use longitudinal data at your organization? Yes or no? If you wouldn't mind sort of responding to this. And then if so, how often is that data queried as part of your, you know, planning, marketing, or consumer analytics? We'll get into this on the next slide. Just as a clarifier here, when we say longitudinal data, what we really mean is a comprehensive data asset that lets you track cohorts of patients chronologically throughout their care journey. I will leave this question up here for just a few more seconds to give everyone a chance to log their responses and then we'll go ahead and discuss the results. All right, just a few more seconds here. All right, so let's jump in and see what the results look like. Pretty split across the board. It does look like though the majority of you, do have access to some form of longitudinal data about 80%, but it looks like there's some variation on how frequently that's utilized, Although a significant portion, looks at the largest sample there, actually does use this data pretty frequently, which is great to see. Across the next few slides, I'd like to highlight the benefits of this type of data for those that aren't already using it, while also hopefully bringing in some new use cases or ideas for folks that may have already incorporated this data into their analytics in some form or another. So a pretty good a moment ago, but just kind of level set on longitudinal data. When we say longitudinal, what are we really talking about here? And broadly longitudinal data, as the name suggests, is data that has the ability to track information across a period of time. Now, when we're talking about longitudinal data in healthcare, that usually means the ability to track individual patients or cohorts of patients throughout the continue of care. Ideally, we would be tracking those patients across the entire length of time that they are covered by a particular health plan. But at a minimum, we want to be able to track distinct journeys through the healthcare system from origin point, whether that's annual physical physician office visit, ED admin, through into a procedure, and then into any sort post-acute care that might follow, all the while also including ancillary and associated services that also relate to this overall journey. Things like lab, and imaging, and prescriptions. Another crucial component to the longitudinal data is that it gives us insights into these patients regardless of care setting. So that includes not just inpatient or hospital-based care that you probably already have some insight into from your state hospital association data or another claim source, but also wanna make sure we're capturing care and claims generated at physician offices, freestanding imaging centers, SNF, home health, things like that. These additional care settings ensure that we have a true understanding of the entire treatment pathway for these patients. And traditionally, we also wanna be able to chart that journey in multiple directions. So whether that is segmenting care that happens before and after some key procedure that kind of serves as our center point in that journey, or looking at it from one direction or another where we can instead begin with, you know, the point of a specific diagnosis code, and say from the point of that specific diagnosis on a patient, what did their treatment pathway look like? What sort of specialties did they see? What types of procedures did they have done? So that we can look for the trends down from that specific diagnosis. You can also see the call-out here on the right-hand side of the slide, but I think it's worth mentioning, not all longitudinal data is truly longitudinal. To really have longitudinal data we can't have any gaps in an individual patient's coverage that might skewer our analysis by missing certain types of procedures or claims generated in certain care settings. I think you'll also wanna be careful about any potential modeling or sampling errors that might be a part of this type of data asset. We'll go into more detail regarding why on the next slide, but in general, longitudinal data is less likely to be a representative sample of your entire market. And so it is typically best for more targeted analyses as opposed to trying to extrapolate trends for the entire market. So with that basic understanding in place, wanted to spend some time kind of comparing and contrasting the most common types of data sets that are available in the market. This slide here is indicative of the two data assets that I personally use most often here at Optum, but my guess is this will generally feel familiar to many of you as far as the most commonly available data sets that are, you're potentially using in your day-to-day. So we'll start on the left hand side of the slide here with the all payer claims data. This is the data that we have had the longest at Optum, and my guess is that most of you have probably worked with some form of this data yourselves. This data can come from a couple of different sources, but it's traditionally gathered via what we call a clearinghouse model. At Optum, we work with LexisNexis is our primary source and they essentially serve as a data aggregator connecting with a number of different claim switches, clearinghouses, and processors that all give them the ability to craft a data set that is both representative of all payers in any given market, but also data that is consistent across markets, so that we don't have to make adjustments or have any coverage gaps as we move across geographies. Or if you happen to, you know, cross state lines as part of a given analysis. This type of data is usually considered physician-centric as a way to analyze the market. The provider level detail is really strong, even if some of the components of the patient data are potentially redacted based on the nature of how these claims are collected via that clearinghouse method. We'll get into more detail on the next slide, but broadly I would say that all payer data is best served for use cases that relate to market share and market sizing, whether that's the book of business for a given provider, or specific competitor facility in your market, or whether you're just trying to prioritize demand for certain services. Because the all payer data is more likely to have stronger coverage rates and it really does have that all payer representation, this data is the best lens into the truest denominator of care that is performed within a given market. So to move over to the right-hand side of the slide and compare that with available longitudinal claims data offerings. Now this data asset is, as we just discussed, more of a sort of patient-centric data asset. These claims are largely concerned with the ability to monitor individual patients or cohorts of patients as they move throughout their healthcare journey. Longitudinal data sets are often built slightly differently as they aren't traditionally sourced via that clearinghouse model, but instead either come directly from a payer source themselves or at least through an organization that has some sort of close tie to a payer or a group of payers. So you will see some variation kind of vendor to vendor in terms of which payers each organization has access to in their longitudinal data. This does also limit the data asset in its overall coverage, which kind of represented here on this slide by a slightly smaller circle as it's part of the Venn diagram, is that means that longitudinal data is typically only available from payers that are willing and able to sell that data into the market. Across the last couple of years, we have seen that number grow, but there are certainly still some payers that haven't yet made their data available with this level of granularity. And so one of the things that, that means is that coverage is not consistent necessarily across all markets. It depends a little bit on the presence that each of those individual sources has within any given market and the size of their patient panel within any sort of given community. So a clearinghouse model is a little different in the sense that it happens to catch most claims for any patients that reside within a given market of interest, whereas longitudinal data is more binary. A patient either is or is not included, depending on whether they're covered by one of the insurers that feeds into your data asset. And if they are in your data asset, you know, you have complete coverage of their entire treatment journey across that time period. But so again, that does mean that coverage can vary a little bit market to market depending on the coverage for those lives. So for example, at Optum, based on our longitudinal data sources, we've got about 40% of the US population covered in our longitudinal data assets. But that coverage can vary from as high as 70% in some states to as low as 20% in others, just depending on how much of each market is controlled by the payers that we have contracts with. And so I think this is kind of a primary consideration for your use of the longitudinal data, making sure you understand what coverage looks like in your individual market, which sources feed the data into any individual vendors that you might be considering for partnership. And that's also why, as we talked about earlier, you want to use a little bit of caution in terms of doing any modeling or forecasting on this data as these data sets are not built out of representative samples of the market and the patients that are covered by the payers that you have access to don't necessarily react in the same way, or follow the same facility, or physician referral pathways as those that might be on a different insurer. And so this is why we still rely pretty heavily on the all payer data for any analysis where we want to size the market appropriately. At Optum, we tend to lean a little bit more conservative in our data approach. So we don't do any forecasting or modeling on top of our longitudinal data. But you'll kind of have to make the right decision for your organization as to your level of comfort with any particular modeling, or whether you feel like coverage rates for this type of data set were high enough in your market to be valid for any particular use case. Now the good news is that while coverage for longitudinal data is often lower than what you see in the all payer data set, that lesser coverage is balanced out with additional detail and granularity that we can get from these data assets. So not only are we getting more comprehensive claims for an individual patient, but that data is also supplemented by demographic details like patient age, gender, or their zip code of residence, and things like that allow us to create much more targeted analysis on any given sort of market of interest. So now that we've talked about how those data assets differ, we wanted to drill a little bit more into which use cases we think are a fit for either data asset. It's important to point out that while, you know, we at Optum use both of these data assets as part of our claims analytics platforms, we don't really combine them in any way in our systems. These data sets serve a similar purpose, but they can also be a little bit apples and oranges in terms of the fields that we get access to on a claim, or data refresh rates and time lags, or consistency of sampling within a given market. And so that's why from our perspective, it's not really a question of like, whether one of these data assets is valuable so much as it is about which of these two types of data is the best fit for the specific question that I'm trying to answer within any individual analysis. So this slide here is a listing of some common use cases compared across both the all payer and longitudinal claims data sets. And the Harvey balls for each of these use cases are essentially a representation of how well each data set aligns with a particular use case. So the more of each circle that is filled in, in each of these instances, the better fit that data is for a specific use case. You can see that some of these use cases are served in some capacity by both of the data assets, but there are pros and cons for each. So, for example, in that first row for like market share statistics, the all payer data is best served to understand your capture of the market, since the all payer data holds that truest representation of the entire patient population within a given community. But there is still some benefits using longitudinal claims in that you can understand your share of the market as it relates to the payers that participate within your longitudinal data asset. Now that won't represent all payers in the market, but that might be the one, the angle that you want to take if you are specifically looking to target a payer with whom you have a favorable reimbursement rate, or maybe you already have a partnership with that payer. I think the same is true for understanding referral networks or anything related to specific providers. You should be asking yourself what is more important in this situation for a specific analysis? Am I trying to have a comprehensive understanding of their entire book of business, or is it more interesting for me in this case to see can I get an understanding of their procedural and referral patterns as it relates to specific payers or specific payer categories? Moving a little further down this list, we'll shift our focus a bit to the use cases that are best served coming from the longitudinal data. Most specifically, some of these things like longer term patient journey tracking or share of wallet analysis. In this case, I think the longitudinal data is really critical to an accurate analysis there, as we need to ensure that we have complete claims coverage for each patient in order to get a true understanding of the percentage of their healthcare spend that is happening, you know, within our networks. And so we will get into more detail here later in the presentation, but if I'm trying to understand our network integrity, or our share of wallet, for example, our orthopedic patient population, a data asset that might have coverage gaps in, you know, free standing physical therapy, or rehab locations could fundamentally skew my perception of the relationship that my organization has with these patients. So I think leaning on the longitudinal data and knowing that I am seeing all touchpoints with that patient across a longer period of time and without any gaps at specific sites of care, ensures more accurate understandings of our organizational strengths and weaknesses throughout that care journey, and will help me understand where to make adjustments in our service offerings or physician partnerships within each market to most directly address those concerns. So wanted to spend some time kind of level setting on these claim types and how often they're most used in the market. We'll come back to the longitudinal data a little bit later on in the presentation as we bring in more specific real-world examples that will hopefully provide some more context around what we've just discussed. But before we get into that, I wanted to turn things back over to Brian who will move us into a discussion of some of the consumer-centric data assets and how those compliment some of the pieces that we've just discussed. So Brian, I'll kick things over to you.
[Brian]
Yeah, thanks Nick. So like Nick kind of just summaried, you know, Optum has been working with the physician-centric all payer data asset for over a decade. And so, you know, we've done a lot of work in that space. One of our newer investments, obviously, is the longitudinal patient-centric data. We've been working within that data asset for a number of years. So we've gotten some reps and some experience working with that data asset and in compiling that asset. One of the other newer, newer compared to the all payer, I guess, but one of the newer assets that we've invested in heavily as well is the consumer-centric data, which, you know, is something that gives you a little bit different lens into both your market and into a different profile of potential patient. And so we wanted to kind of go through and talk through a little bit about the consumer data assets and what benefit that can bring to your organization as well. So using consumer data allows you to combine a lot of the clinical insights that you may be catching within your EMR, or, you know, health record that you're capturing internally, along with key demographic elements outlined that you can see here on the right of the screen. So being able to kind of take the information that you're compiling with your clinical records, and then being able to combine that and understand things like predicted health needs through propensity models, things like payer status, and commercial and financial, consumer financial fields like different insurance profiles, household income, consumers' credit history. Things like demographic and lifestyle fields that really kind of show you household makeup, things like interests, leisure, and physical activities combined with healthcare preferences and then healthcare attitudes as well. So, you know, what are the things that drive consumers to seek out healthcare? There are people who are more engaged in their healthcare journey versus people who are less engaged. And then finally, communication and media preferences. You know, I know for myself, I consume data a certain way. I consume information a certain way that's gonna be different than how Nick consumes data, which is different than how you all do. And so when you have data assets that can give you insights into how consumers want to actually consume a message, it allows you to much better target those consumers in a way that they'll actually absorb it and do something about it. And so those are all different elements of what a good consumer data asset provides. Now at Optum, like I said, we've been heavily investing in both our claims data, our longitudinal data asset, as well as our consumer data asset. We have probably the largest healthcare data asset in the United States at this point when you combine all of those elements together, which gives us a just a war chest of information that our teams can sift through, and build, and unlock insights from. And so when we're talking about a good consumer data asset, it really needs to allow you first and foremost access to all the consumers in a given market. So when I'm looking at my market, whatever that may be, that may be a whole state, that may be a greater metropolitan area, that may be a large rural swath of land, whatever that is, having the flexibility to understand and kind of segment specific geographies is really important. So when we start from a place of total coverage, which allows you to start to segment information based on other factors, that flexibility helps a ton. So I, if I wanna look at my primary service area, if I wanna look at my primary and secondary service area, or if I wanna look at a more tertiary look of it, look at the market, having that flexibility is really important. Then you kind of start to incorporate other elements of the data, or other elements of what the consumer data can provide as well. So things like who will most need or is most likely to need care based on whatever given service line or sub-service line that you're looking at. It may also not just be service line focused. Maybe you want to understand, you know, who are the people who are gonna seek out care in my ED? Who are the people who are gonna seek out care in urgent care facilities? Who are people who are most likely gonna need or be eligible for Medicare advantage? Those are all different types of fields that a good consumer data asset will allow you to kind of search through. And then finally, when you start to kind of sift down from a place where you're looking at the whole market down to specific geographies and then specific service line needs, things like that, you really want to understand who are consumers who are likely to engage in their healthcare journey. I think we all have different profiles and we all have different levels that we participate in the healthcare process. You know, for some people like my father, he doesn't want to go to a doctor for anything if he doesn't have to. He's less likely to act on information that he receives about healthcare. For someone like myself, I am much more engaged in my healthcare journey. So if I receive information about screenings or if I receive information about, you know, seeking out information from my primary care physician, I'm much more likely to go, and ask questions, and respond to screening invites or things like that. And so understanding who those people are who are much more likely to engage are gonna only help us from a targeting standpoint. The other thing that we do here at Optum that I really like and that I think helps us navigate, and kind of filter through the consumer data is we have a data science team dedicated to building out things like propensity models. That data science team employs kind of AI, machine learning techniques and habits to really understand, and get a better sense of consumer landscapes, and how combining information from clinical claims data, clinical and claims data into the consumer data asset to really help us understand these consumers and the profiles of how they engage much better. And so, you know, for us that data science team helps, has helped us build out over 120 clinical propensity models that are gonna give us insights into who is most likely to need care when and within, you know, given service lines and sub-service lines. We have over 900 points of data on every one of, every consumer over the age of 18 in the United States. And so for us, having that number of customer attributes allows us to do a lot as it relates to segmentation and again, understanding who these people are. So from a targeting standpoint, we have a very rich and robust ability to kind of look at that data and target down to more specifically who we need to reach right now and who are the potential people that will easily or more easily convert to patients. And then finally all that information really allows us to segment in a much more intelligent way. And so our data assets include custom segments, or include standard segmentation. So gives us a sense of different profiles that certain consumers in the market will fall into. But then it also allows us to work with our clients and our, that we work with to build out custom segmentation. So if you're looking and understand certain profiles of patients that come into your ED on a regular basis and you want to build custom segments around those different consumers, we both have had experience and have built those types of custom segments, as well as through our value management team is able to, are able to dig in and pull out some of those psychosocial indicators that helps us build those segments even better. So it's, you know, it's, there's a lot that goes into a really good consumer data asset, and I know the you, the data alone isn't kind of the end-all be-all. It's really having the team to make sense of it and to be able to help kind of sift through all the different data elements that fall into it because it's really a robust asset. Now for us, you know, everyone's trying to increase market share, but it's really hard work. So there's a lot of competition for your market and that competition's only increasing. While everyone fights to kinda win or retain volumes, all of our costs continue to increase. And so we're all kind of working to grow and we're working to get a larger piece of the pie, but it's certainly not easy work, and there's a lot that goes into it, and a lot that we're kind of dealing with. I know from our standpoint, you know, we look at organizations, we work with organizations, and when we look at kind of their planning departments and strategy. When we look at the organization that kind of falls under business development strategy, typically what we find is that planning, marketing, position outreach, network optimization, they all kind of share broad understanding of growth goals, but very rarely is their execution aligned. Typically we find that it's siloed and uncoordinated at best in how they're actually gonna go after that business. They're usually lacking data to make better choices that helps them to understand the need to align their approaches and their strategies. And ultimately with all these challenges that we are facing externally, we need to work more effectively and efficiently as a team to really achieve what it is that we've been asked to do. And so if we're gonna be successful, we have to kind of both utilize data in a different way, but also utilize our teams in a different way to capitalize on the insights we're getting from that data. You know, for us what's clear is that we can't be successful working in silos with an uncoordinated approach. You know, what we've found is a lot of organizations, you know, will, you know, they'll go after one or two of these different strategies with different parts of their organization or different teams, but very rarely are we finding organizations who are aligning that work in a meaningful way. And so, you know, whether you're developing a strategy to win back market share and you're encouraging providers to reduce out migration, you know, very rarely are we seeing organizations also working on personalizing your precision marketing techniques, or establishing an integrated and enriched data asset and source. So what we know is that if we're gonna be successful, we really need to coordinate our work so that we're all working together and working in a way that both supports each other and other teams, but also compliments the work that going on out there. So, you know, the facts are that by using both the longitudinal data asset along with the consumer data, you can develop a more cohesive growth strategy that will allow you to be much more effective and efficient with your resources. So that's kind of where we see this going in the future and where we see organizations who are much more effective and efficient with the business of growth, and strategy, and business development. Now, when we develop growth strategies, we typically say that we want to grow, you know, things like either ortho or cardiac. So we're developing kind of overarching growth strategies. What we typically don't say is, well, marketing is responsible for growing ortho and physician outreach is responsible for growing cardiac. We think that incorporating an approach where your different growth engines are supporting and complimenting each other will allow you to work much more efficient and effective. So like I said, where we're seeing people be extremely successful is when they're starting to develop a strategic plan that cascades down to the whole growth organization and not just a silo department. So for an organization who has, you know, prioritized, you know, specific growth goals, you know, they may be focused on acquiring new patients, and doing that by targeting consumers and segmenting audiences, then they deploy some sort of precision direct-to-consumer marketing approach to do that, while at the same time really focusing on the providers in their market to really go after that business as well, and retain, and expand services for existing patients, and deploying the physician outreach team. We find that organizations are much more effective at both getting results related to ROI, as well as just being more successful in general when this is done in a coordinated effort. And so if our focus is really around growing orthopedic business, let's have marketing out in the market, you know, putting that message out so that consumers are responding and starting to think through the different ways that they can access orthopedics care within your organization. At the same time, our physician and outreach team should be out in the market talking to both our primary care physicians and our orthopedic surgeons, letting them know and reinforcing the message that the consumers are hearing through the work that marketing's doing. When we do that, what we're doing is we're creating a much more, again, efficient and effective approach at doing this, at going after orthopedic business that is, you know, supporting each other's work so that we're much more effective at achieving the results that we're looking for. You know, we're all looking to do, we're all looking to grow. And I'm sure none of us are in a situation where our leaders aren't asking us to slow down with growth. They're asking us to be much quicker. They're asking us to be much more effective at bringing patients in, in a timely manner. When we do this, when we kind of support our approaches from both a consumer and a physician standpoint, we can do that in a much more timely manner and be much more effective. So we find that these data assets are much more complimentary. And the work that comes out of the insights that you're able to gain from the different data assets, it's a much better approach when that work is complimented from the different departments who are responsible for it. So just a thought and some insights from some of the work that we've been doing out in the market with different customers who have been extremely successful at this approach. All right, with that, I'm gonna turn it back over to Nick to talk through some real-world examples of how using these data assets has been successful.
[Nick]
Great, thanks Brian. So yeah, I wanted to just use the last portion of this presentation to talk through some of those real-world examples and give you a sense of how some of these data assets differ in terms of the strengths and weaknesses of how they're incorporated into the market. One kind of note here is just given the competitive nature of most of these use cases, we have blinded all facility named markets providers that sort of thing were appropriate just so that we have the ability to talk about these examples in more general terms. So with this first use case, this is one that I think most would consider to be kind of a real bread butter use case for claims data. And this is one that I think is best served by leveraging the all payer side of the data asset. And so the story here is that we were working with an organization that wanted to set their growth agenda across the entire system, and that included targeting key procedures that were in demand in their market, but also thinking about specific geographies within their community to understand the competitive landscape and the viability of capturing new business from these markets. So the first step here is to identify the high-value services in their community, and we did that by just looking at the claims for their market and segmenting them by procedure code and facility. So that allowed us to not only value the most highly utilized services in this market, but also this organization's current share of those services and maybe most importantly how much remaining business was still out there in the market. And so that allows us to zero in, not just on those key services, but also think through the logistics of, okay, if we capture 5% or 10% of that remaining business, what is the value of that care to our organization? And does it justify the business expense of acquiring a new provider, or purchasing a new piece of equipment, or adding an outpatient location? Whatever the appropriate strategy that we think is that might have the most positive impact on care within that market. We can also hear as part of this, look at the competitive landscape in that community and see what facilities would we be up against if we were trying to capture that business from this market. Are there trends around, you know, is that competition coming from a hospital, or an ambulatory facility, or is there a mix of both capturing care within that community? Which physicians are the most significant players in that market currently? And so in this example we decided to focus on spinal surgery. And based on what we saw in the data, we also were able to identify that the Northeast corridor of their selected market is the best fit for their overall organizational strategy. And then kind of moving to the right-hand side of the slide, we identified the four physicians in that same market that we think provided their best bet to grow volumes into this new spinal surgery center. And so I think the reason that this is, a use case sort of best fits in the all payer data asset is as we discussed earlier, to sort of truly understand the value of a particular service in a particular market, or to understand the value of these four providers that we've identified. I want the most comprehensive data asset that is going to give me the most accurate size of that market or the book of business for these providers, regardless of payer or other factors, so that I can truly identify the busiest competitor facilities and busiest providers in the market without sample bias. And when I'm looking at referral dynamics or procedural patterns within that market, we know that that is something that is consistent across the entire community that we are analyzing. Now the second use case is interesting because this is one that I think actually benefits from a combination of both all payer and longitudinal claims, sort of tackling the same problem through different lenses. This case study is one that's focused on identifying the right location in which to open an ambulatory facility in our client's secondary market. This was a hospital that had a presence within a city center and they were looking to kind of build out their ambulatory locations in the surrounding suburbs. So the first thing that we looked at is very similar to the last example, wanted to understand the competitive landscape in that market, the physicians that are active in the community, and then what the referral dynamics between, you know, primary care providers and surgeons looks like within that community currently. But we also wanted to supplement that analysis with some more detailed understanding of patient origin, which is going to come from the longitudinal data sources. And so this is where I would want to understand where patients are being referred for these procedures actually reside. Are they being seen close to home? Are they being referred out of market for care that we think we would've had a shot at capturing if those services were made available in a closer proximity to where these folks actually reside or live? And so the patient origin data becomes a really valuable piece of this puzzle as we look to better understand the impact that geography plays on referral patterns or facility utilization within a market. Now, I would've said like those data points were valuable regardless, but I think it's worth mentioning that we have seen a massive spike in utilization for that patient origin data from our client base across the last two or three years with a good chunk of that really being driven by COVID. Not so much the treatment of COVID as it is a recognition of the fact that the COVID shifted so many industries to a work from home or at least a hybrid model. And so in markets where patients maybe lived in suburb X, but worked in urban city Y, that likely played a role in where they engaged with healthcare. If that patient no longer commutes into the city five days a week for work, that probably also has an influence on which markets they're prioritizing for healthcare. Maybe not for inpatient care, more complex procedures, but certainly for outpatient or ambulatory services, like we are discussing here, especially, in a market like, for this client where they had that urban center for their hospitals that didn't have much of that presence in the suburban markets. So this idea of understanding where these patients actually reside, what the availability of care looks like in those markets currently, and how that might influence physician or facility choice made the longitudinal data a real value add to this specific type of analysis. One other piece to add here too is in addition to looking at the strategy across both types of data, the other thing that I think is important to look at is to make sure we are also kind of trending and monitoring that data across time. So beyond individual patient journeys, what are those trends looking like across a, you know, six or 12-month period or maybe even longer. Are patient volumes increasing and decreasing over time in those communities? Are the referrals that this, you know, ambulatory facility generates for our acute care facilities moving in the direction that we would expect? I think that piece is critical when it comes to making, you know, downstream revenue or ROI calculations to help us evaluate the success or failure of these given initiatives. And having the claims data to supplement your internal staff will help provide some additional context so that we sort of know we have that full understanding of the market. For example, if our internal data shows, you know, patient inflow from that market was up by 5%, that's a positive trend. But if broader market data shows that all of the referrals coming out of that community also grew by 10% during that same time period, that changes my assessment of our strategy here because even though our raw volume of patients is up, our share of the overall pie is decreasing. And so that again sort of changes my perspective on the success of this project, but it's also not something that I would necessarily be able to suss out by solely relying on our internal statistics. In use of time here, I'm actually gonna skip over this next use case, you can refer to it later if you're interested. This one focuses on kind of using the claims data for physician acquisition strategies and how we kind of value partnering with the right practices, but also sometimes just as importantly, how do we value the benefit of kind of avoiding partnering with the wrong practices for providers. But wanted to save a little bit of time to actually sort of in the last use case for me before we move to the consumer data is one that focuses solely in the longitudinal space and one that I think really requires a longitudinal data asset to understand. This case study focuses on surfacing weak spots along particular patient journeys. This dovetails in with some of that patient share of wallet stuff that we discussed earlier. In this instance we were working with a client that had a pretty good sense of their market share for sports medicine, but market share without a sort of full sense of all of the claims that patient generates, is sometimes really sort of more accurately a share of surgeries or a procedure share as opposed to it as sort of an actual share that you have for that that patient in their entire journey. When you're talking about orthopedic market share, you're probably talking about your share of hips and knees, actual surgeries, things like that. But you wanna make sure you're not missing upstream business. So for example, presurgical imaging or labs, or maybe you're missing post-surgical care like rehab. And so longitudinal data really flips this idea of market share on its head a bit, in the sense that we were talking about our share of the entire journey for these orthopedic patients, especially useful in pre and post-acute care settings as there's often more competition for those services than there are, you know, the number of available facilities that are offering, you know, inpatient surgical care. And again, those instances, those pre and post-acute elements are also where patient geography might be a more significant driving factor in the choice to, you know, visit that freestanding imaging center, or the outpatient rehab location that is potentially closer to the patient's home, but not necessarily a part of your network. So if you look at the two pie charts on the left-hand side of the slide, what's interesting here is that you can see a pretty significant delta between the organization's procedural share and their share of the entire journey, which is encompassing a 90-day window on either side of the actual procedure. So the share of sports medicine procedures is 70%, which is pretty good, but their overall journey share is only about 45%, meaning patients are flowing in and out of their networks throughout the course of that journey. So as we move to the columns here, towards the center of the slide, you can see their share of the procedure itself, which is that first column is strong, but how that then cascades into rehab and PT, potential readmissions, physician fees, imaging lab, everything included there, and we can break that share out separately. So the darker portion of each of these columns is representative of care done at this specific client's network. The lighter portion is work that is done elsewhere in the market. And so what you see here is while their share's pretty good across most of these elements of the journey are at least consistent, the real weak spot here was in rehab where the lion share was being done outside of their network. So we can drill into more detail here to see what that weakness is potentially driven by. Are their geographic commonalities within their patient population or maybe it's an issue with, you know, downstream referrals from individual physicians, or physician practices that are utilizing these competitor PT locations? But this data will help us determine where to drill deeper to try to understand what levers we need to adjust, kind of stem some of that out of network migration. The other thing I like about this analysis is that when we get into the competitive landscape and some of these share statistics, this is not an assessment of the physical therapy market at large, but it would instead be trying to understand physical therapy that not only follows a specific in the sports medicine procedure, but that follows a procedure done at one of our locations. So this is analyzing a smaller slice of the market, but in theory that should be more actionable as we are only looking for opportunity within a patient panel with which we already had some sort of primary touchpoint. So I think this helps us avoid overstating opportunity in the market because we aren't including, you know, PT done on a patient that was seen by a competitor for their entire ortho journey. And again, we could be even more targeted by refining that population down to see are their broader trends based on gender or age, or perhaps there are geographic elements to this based on where the patients live that might explain their facility choice or their referral patterns. And so these are the kinds of insights that I think are available when you have all of the stages of a healthcare journey at your fingertips, and I think it makes a strong case for the value of longitudinal data in these situations and a lot of these sort of patient-centric components to the analysis. All right Brian, let me take this opportunity to kick things back over to you. Do you wanna walk us through the last use case here?
[Brian]
Yeah, that's great, thanks Nick. So with this use case what we're really looking at is using the consumer data and mapping breast cancer access points in the Philadelphia market over a three-year period. And so what this organization was really focused on was narrowing the population. So we started looking at female patients over the age of 45 where we were able to kind of get, start that at about 350,000 consumers who kind of fit that description when we started to kind of, you know, wean that down further, we were able to identify 344,000 screening mammographies, 50,000 diagnostic mammographies, and then really start to understand, and see the patients diagnosed with breast cancer, which was really focused around about 4,600 patients. When we did that, we were able to then kind of, for this organization identify 1000 patients that received radiation therapy, 950 that received chemotherapy, and over 2000 that had a surgery performed. So for this organization, over a three-year period saw significant increase in breast cancer related procedures, and diagnostics, and treatments within their patient population. And it was all because of, it was all because they were able to identify and segment those patients with a message at a much more detailed and deeper level so wildly successful for this organization. With that, we have about 10 minutes and I've noticed that we have a number of questions on the line. And Nick, I was gonna kind of direct a couple of them towards you 'cause a lot of them have to do with the longitudinal data asset. And so maybe if I could-
Yeah, sure.
Kind of cue you up, I'll kind of read some of them off here. And just so you know, if we don't get to your question here on the call, we will definitely reach out after the fact, and make sure that we get you some answers related to what your question was then. So please don't fret if you don't get your question answered. So the first one, longitudinal data still sounds like claims data based. Are there other components to it that are beyond claims, like clinical details, notes, vitals, lab results, et cetera?
[Nick]
Yeah, I think, broadly longitudinal data still is claims data based. And I think that is likely to remain for quite a while in the sense that, that is the data that is more publicly available, or can be sourced and shared directly through those payer sources. I think the incorporation of some of those clinical details and like lab results, and quality elements, and things of that nature are traditionally done on a more one-off basis. We do some work at Optum with that where we take, you know, records from a particular client's EMR and combine some of that with what we're seeing in the claims data to round out some of our analysis there to see what trends that drives. I think the difficulty is there aren't great national sources to be able to capture that data so that we kind of lose insight on what's happening for patients at competitor locations. And I think it's gonna be difficult to acquire data sets that give us much of that detail. I think we can use some of what we're getting from like EMR systems in particular markets to develop some sort of benchmarks, but a lot of that detail is sort of sensitive to the individual patients and it's just not as publicly available as the details around the physicians that they've seen and procedures they've had done, and things like that.
[Brian]
Great. Nick, another question. What's the biggest hurdle preventing longitudinal claims from matching the coverage figures seen in claim sets sourced from clearinghouses?
[Nick]
Yeah, I think it's, you know, in a clearinghouse model all of the individual, you know, processors and switches have the rights to sell those claims as an additional revenue source. And so there are a number of different sources that you could go to build out an all payer claims data asset that are still going to have pretty comprehensive coverage. Whereas on the longitudinal side of the data, it really does kinda have to come from the horse's mouth, if you will. So there are limitations to essentially just the number of payer or primary sources that are willing to sell that data out into the market. I think it's something that we have seen grow across the last couple of years and my guess is it's going to continue to grow across the coming years, but again, sort of, a bit at the mercy of those primary sources and their willingness to share that data.
[Brian]
One more Nick, and this one was, this one was specific to I think the last case study that you walked through. It says you're talking about share of wallet from a revenue standpoint of view but not profit. I wonder, I think that one's just, can you explain that?
[Nick]
Yeah, I think, you know, from our perspective we're looking at that a little bit more from a revenue point of view as opposed to the profit side, simply because like in these examples we are are looking at the value of care being performed across multiple care settings and potentially across multiple networks. And so a revenue stat is a little bit more easily normalized in terms of having the level of detail for reimbursement rates for, you know, benchmarks across specific procedures at specific facilities. Where I think the profit piece is a little bit more, more complicated to get into, especially, you know, for the piece done at competitor locations. We obviously don't always have the financial detail of what, some of that care was worth to our competitors. And then I think there's also some difference internally around, you know, profit levels of care done at a hospital setting versus an ambulatory setting. And so I think the top level of that analysis is just, okay, what is it worth to us if we capture X procedure or Y procedure? But I do think there's value in sort of a second step of that analysis to determine the profitability of that care in terms of the setting where that care was performed, where specific procedures fall along that journey, what other ancillary associated services happen if we make treatment decision X or Y that influence profitability for those referrals and for those patterns that I think are absolutely worth considering.
[Brian]
Great. And another question, and this one is more for me, this is for the breast cancer use case. Can you explain how data helped them improve their volumes? Yeah, in fact I should have explained it better. So one piece of that is exposing the opportunities within the consumers in the market. And so they used the data to kind of, the first thing they did was they overlaid our propensity model, which again is built by our data science team to really understand who are the consumers in the market, who are, you know, most at risk, or most likely to need care for breast cancer treatment over the next six to 12 months. What we do with our propensity models is we score every consumer in the market on a scale of one to 10, and so one being the least likely to need care for breast cancer, 10 being the most likely to need care over the coming year to breast cancer. And when we do that, that's where they came up with the 354,000 females over the age of 45 who, I think they looked at the nines and 10s on that scale as far as how they started that. How they actually kind of whittled that down even further and how they acted on it was once they identified the consumers, then the marketing campaign really kicked in. And so our value management team, along with the organization's marketing department, and marketing agency, or advertising agency started a digital marketing campaign targeting those specific consumers, giving them messages about the screening mammographies, trying to get them in for those screenings. And then identifying who further needed care through diagnostic mammographies, which is where, and how they were able to get those 4,600 new patients in the door. So it was, you know, it was really through the data that they developed the plan and the target list, and then it was through a precision marketing campaign, really kind of done by both the, our, you know, the organization's marketing apparatus, their advertising agency, as well as our value management team to execute on a message to those consumers to really get them in the door. So that's really kind of how that worked out. I know we have some additional questions on the line. I think we will probably need to reach out to y'all and follow up to make sure we get you answers. We wanted to thank you all for attending, and really thank you for your time, and listening to kind of us talk through the different types of data assets that are out there, and kind of our spin on it. Nick, I don't know if there's anything else, any other information we wanna make sure that we share before we kind of close up shop here.
[Heather]
Yes, thank you to our presenters today for sharing your insight and expertise with us. I would also like to thank you, our audience, for sharing your valuable time. We know you're all busy and we appreciate your attendance. Please take a moment to complete the survey and let us know how we did today. The survey is located within the survey widget on your audience console below the slide deck. Also, this presentation will be made available on demand within approximately 24 hours of this live webinar broadcast. The on-demand presentation can be accessed using the same audience link that you used to enter this live broadcast. We just wanna thank you again for joining and have a great day.
Remain a provider of choice by taking meaningful action
There’s no shortage of data available to track patients longitudinally. But to take meaningful action, this data needs to be aggregated, normalized and analyzed — which can be a vastly different process from organization to organization.
Watch the webinar to learn:
- What longitudinal data is and why you should use it in your growth strategy
- Common misconceptions about longitudinal data and what you should look for to help your organization succeed
- Real-world examples of how leading organizations use this data in their growth strategies
Learn how Optum can support your growth priorities
Related content
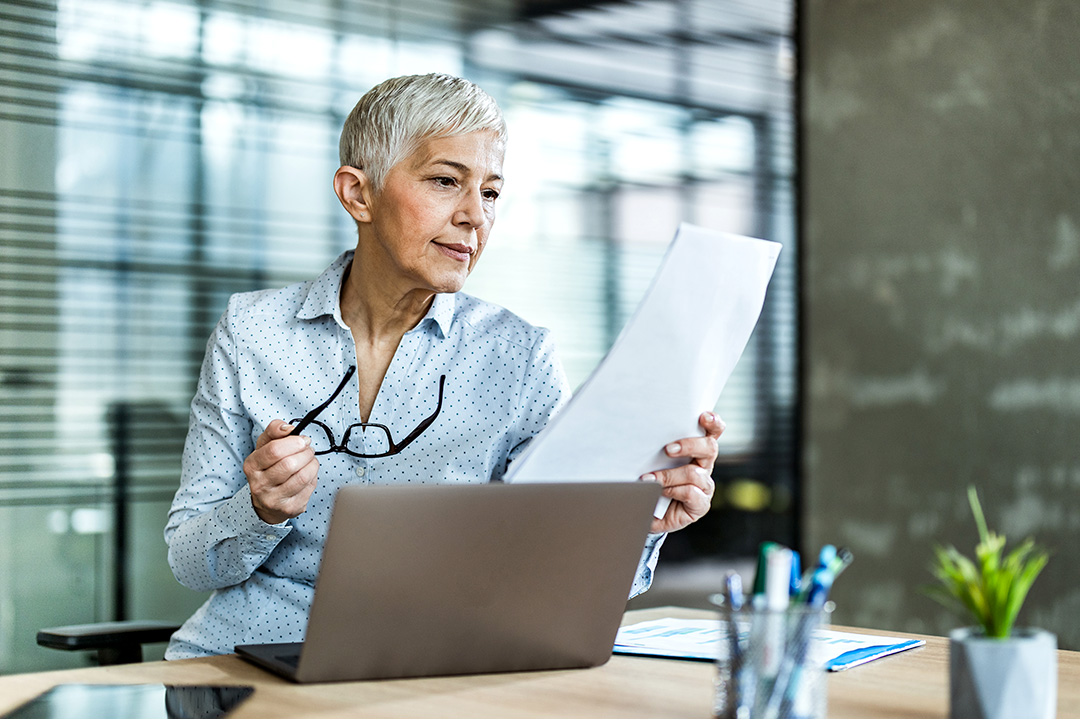
Infographic
Your roadmap for driving growth
Explore 2 growth strategy paths for your organization.
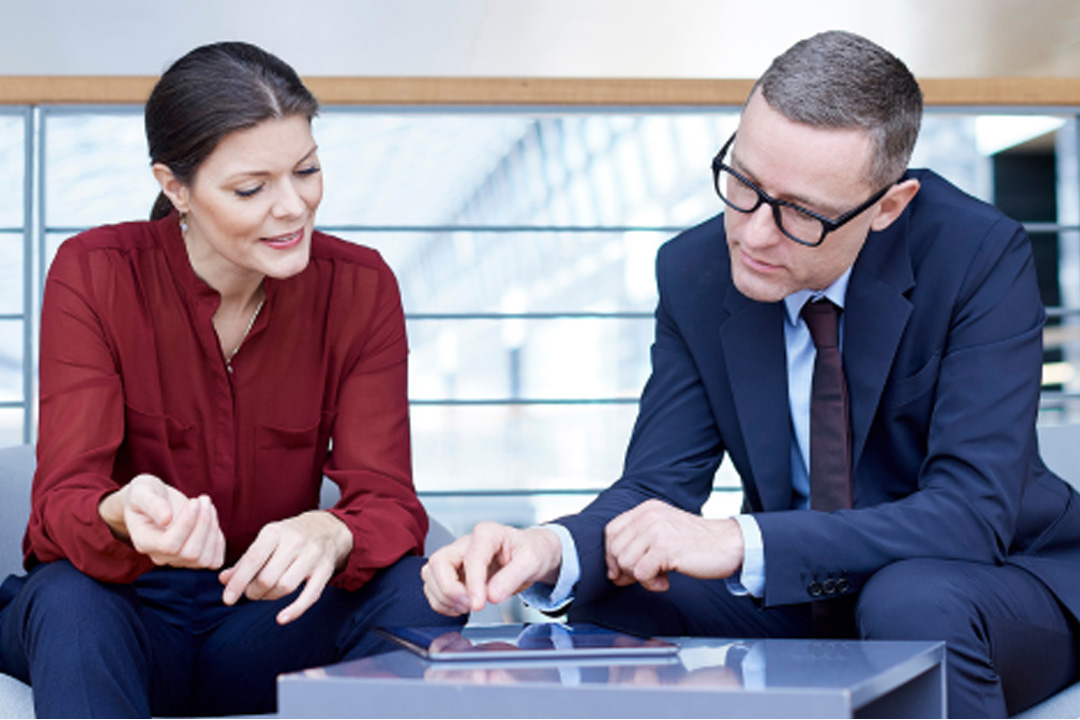
Trends report
Growth trends for patient-centric health care
Read the trends report to explore how you can prepare for growth in 6 key areas.
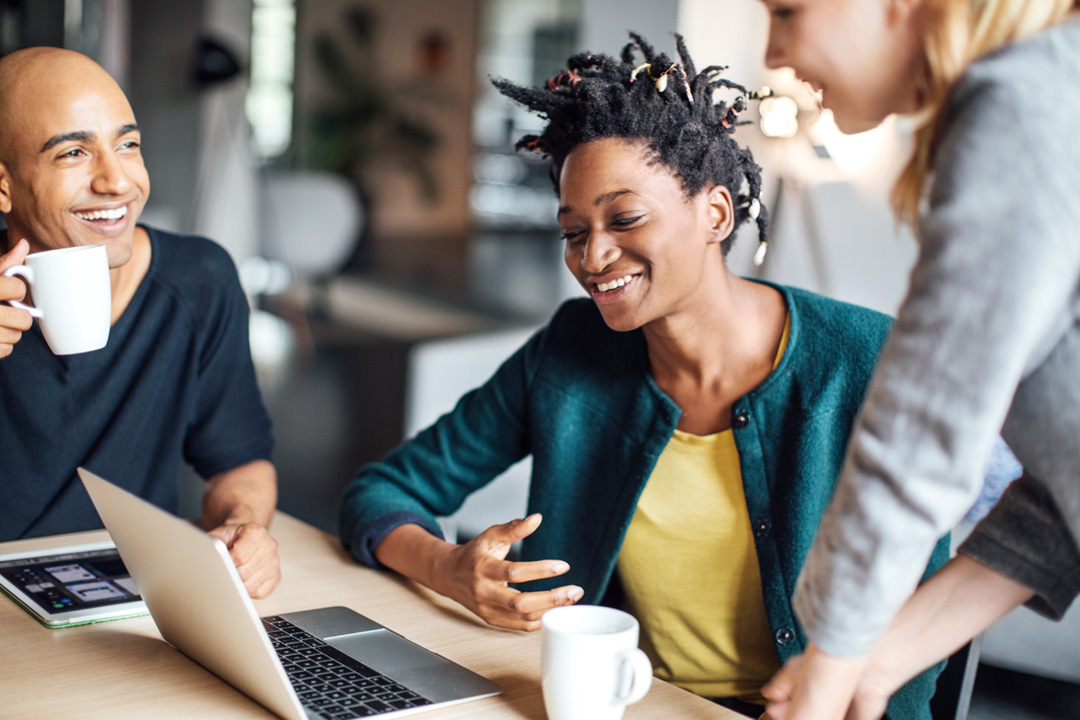
E-book
Driving hospital and health system growth
Learn best practices for business strategies to drive patient-centered growth.