Four key advantages of fully leveraged AI
Hospitals need the right utilization review (UR) technology to secure all appropriate revenue. Criteria-based systems — such as first-level review systems and platforms that use artificial intelligence (AI) in less advanced ways — rely on fixed rules that aren’t developed with physician advisor reviews in mind.
On the other hand, a fully leveraged AI solution designed specifically for case status reviews incorporates deep AI and comprehensive evidence-based research to offer a more accurate and efficient UR process.
Criteria-based systems can support UR, but they aren’t a sufficient basis for a complete UR process. For example, approximately 4 million times, Optum physician advisors supporting clients have recommended a different inpatient/outpatient status than that identified by criteria-based review systems and processes.
Fully leveraged AI solutions are superior to traditional systems in four key ways.

Understand physician meaning
Criteria-based UR systems depend on statistics and discrete rules, but effective UR requires understanding physician judgement in documentation. Between 60% to 70% of clinical information within a patient record resides in the clinical text. Criteria-based systems can have difficulty understanding the intent behind this unstructured data.
Some criteria-based systems are beginning to use AI as a glorified word search tool, but many such tools aren’t sophisticated enough for the job. They may recognize the terms “history of smoking” or “chest pain” in patient records, but they aren’t designed to understand the context.
Fully leveraged AI incorporates more sophisticated and advanced technology. It interprets the meaning of physician documentation, and puts key terms found by AI into context. It applies bi-directional processing methodology that recognizes key phrases and considers them in relation to high-risk factors and low-risk factors along with how they’re used linguistically.
For example, a fully leveraged AI solution would recognize that “no reported history of smoking” indicates an absence of smoking. But criteria-based systems are likely to see “history of smoking” and conclude that the patient smokes.
Learn from past reviews
Many criteria-based systems were designed for basic first-level sorting. They search for clinical high-risk factors and compare them against a set of rules to identify cases whose medical necessity is clearly either inpatient or outpatient.
However, they are limited and aren’t designed to address more complex “gray cases” with ambiguous patient status, such as cases involving dehydration, chest pain or lengths of stay less than two days. Many payer medical necessity denials come from these gray cases.
Fully leveraged AI addresses this gap by learning from the past.
By reviewing thousands or millions of physician advisor reviews, it learns to recognize the nuances of both high- and low-risk factors to better interpret physician intent for these gray cases. This results in more accurate hospital patient status determinations that lead to stronger revenue integrity and fewer denials compared to criteria-based systems.
Self-improve
While some criteria-based systems use AI to search patient records for key clinical data, they rely upon specific, predetermined rules individually coded by a software developer. Fully leveraged AI solutions leverage deep neural networks to improve themselves by reviewing prior physician advisor reviews.
Each time new reviews are fed into the AI system, it uses patterns it recognizes to identify new guidelines on its own, becoming smarter. As a result, fully leveraged AI enables physician advisor reviews based on patterns developed and validated from real medical scenarios, not a programmer’s set of rules.
Support a full UR process
Unlike criteria-based systems, fully leveraged AI accelerates and improves both initial case sorting and physician advisor reviews.
Such AI can find and deliver key facts from patient records to physician advisors, eliminating the need to pour through countless screens and pages. The extensive medical knowledge fully leveraged AI is built upon can further increase defensibility with payers.
This same AI functionality can also help physician advisors overturn concurrent denials during peer-to-peer reviews with payer medical directors and provide a foundation for appeal staff to craft retrospective appeal arguments.
By supporting patient status both up front and after submission to payers, fully leveraged AI strengthens revenue integrity far more than the limited contribution of criteria-based systems.
Related content
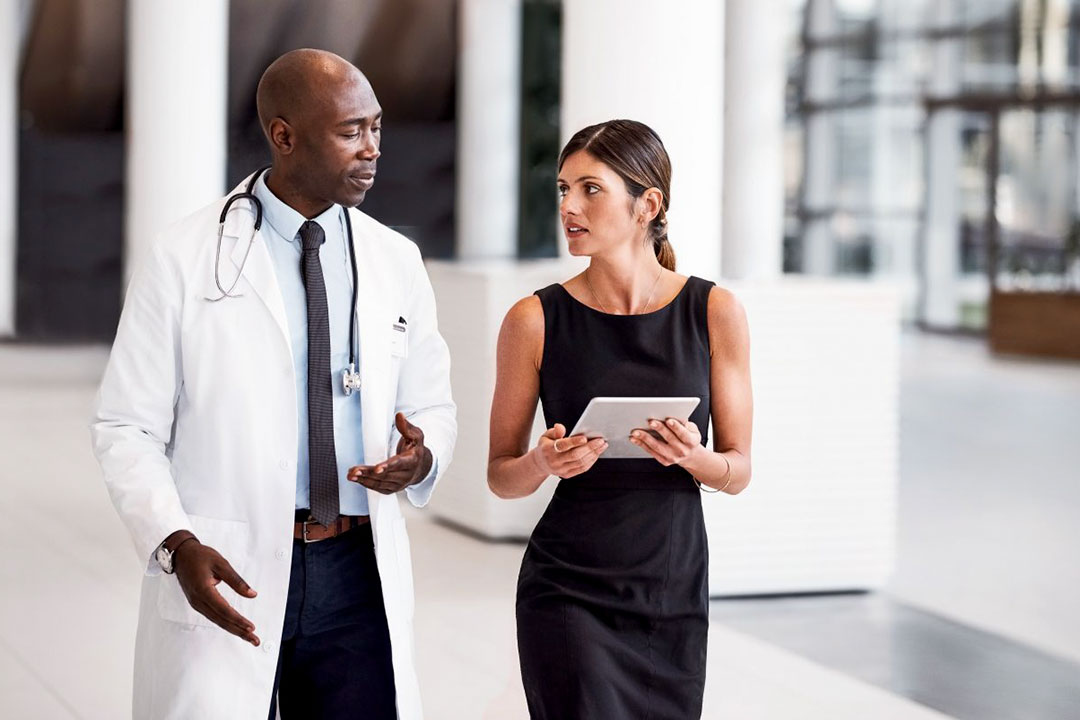
White paper
4 elements of physician advisor success
Explore the elements hospitals need to provide for maximized effectiveness.
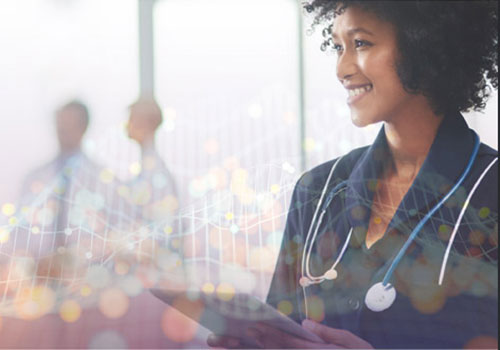
White paper
Disruptive events make traditional UR models obsolete
Learn five ways to use new processes and technology to improve UR.